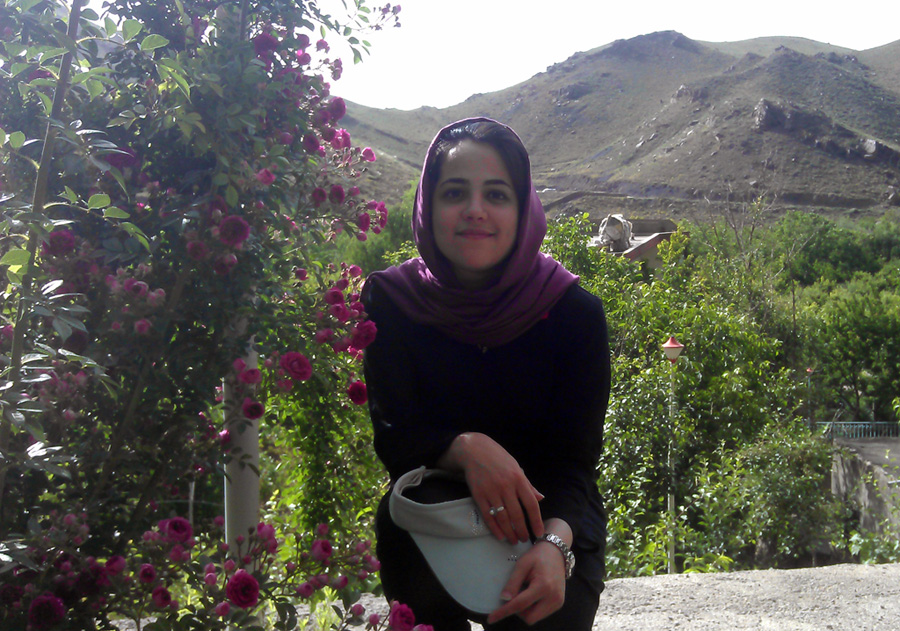
|
Hanieh Poostchi
Master of Artificial Intelligence
|
Email:
This e-mail address is being protected from spambots. You need JavaScript enabled to view it
,
This e-mail address is being protected from spambots. You need JavaScript enabled to view it
,
This e-mail address is being protected from spambots. You need JavaScript enabled to view it
|
Advisor: Mohammad-R. Akbarzadeh-T., Full Professor of Electrical and Computer Engineering Departments, Ferdowsi University of Mashhad, Mashhad, Iran.
Co-Advisor: Seyed Mahmoud Taheri, Associated Professor at Department of Mathematical Sciences, Isfahan University of Technology, Isfahan, Iran.
Master Thesis:
A Novel Framework for Distributed Data Association in WSNs Based on Voronoi Tessellation
Data Association (DA) is one of fundamental phases of multi-target multi-sensor tracking in Wireless Sensor Networks (WSNs). This inference problem tries to find the most probable association between sensors' measurements and target tracks from all the possible associations. A Distributed approach to DA (DDA) can bring better scalability, robustness to sensor failure, and energy efficiency by harvesting the interactions among local sensors for collaborative learning. However, there are two key challenges to DDA in terms of finding a suitable infrastructure that reflects the network topology as a graph and then extracting a spanning tree from it to apply the max-product algorithm. In this thesis, we propose the use of Voronoi Tessellation for a distributed adaptive spanning tree based on Markov property interpretation in WSNs. This tree efficiently maps the network into a Markov Random Field. In contrast to existing approaches, the proposed architecture can be set upon any arbitrary random dispersion of sensors. Moreover, by proposing a new energy efficient coverage cost, we extend the proposed approach to a hierarchical form which results in noise reduction of sensors' measurements and improved accuracy.The utility of the proposed interaction architecture is also illustrated by applying the hierarchical approach for data gathering task in WSNs. The results show that the underlying infrastructure scales well with network properties, prolongs network life and balances the load among sensors.
|
|
|
|
|